Brave New Frontiers: Can computers teach themselves?
Artificial intelligence seems to be everywhere, but what we are really witnessing is a supervised-learning revolution: We teach computers to see patterns, much as we teach children to read.;
By : migrator
Update:2020-04-15 10:21 IST
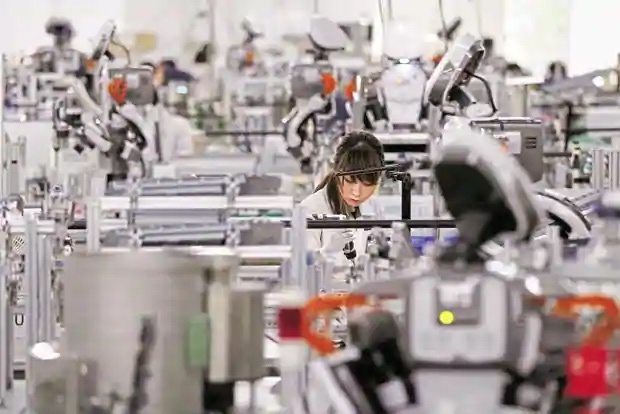
Chennai
But the future of AI depends on computer systems that learn on their own, without supervision, researchers say. When a mother points to a dog and tells her baby, “Look at the doggy,” the child learns what to call the furry four-legged friends. That is supervised learning.
But when that baby stands and stumbles, again and again, until she can walk, that is something else. Computers are the same. Just as humans learn mostly through observation or trial and error, computers will have to go beyond supervised learning to reach the holy grail of human-level intelligence.
“We want to move from systems that require lots of human knowledge and human hand engineering” toward “increasingly more and more autonomous systems,” said David Cox, IBM Director of the MIT-IBM Watson AI Lab. Even if a supervised learning system read all the books in the world, he noted, it would still lack human-level intelligence because so much of our knowledge is never written down.
Supervised learning depends on annotated data: images, audio or text that is painstakingly labelled by hordes of workers. They circle people or outline bicycles on pictures of street traffic. The labelled data is fed to computer algorithms, teaching the algorithms what to look for.
After ingesting millions of labelled images, the algorithms become expert at recognizing what they have been taught to see. But supervised learning is constrained to relatively narrow domains defined largely by the training data.
“There is a limit to what you can apply supervised learning to today due to the fact that you need a lot of labelled data,” said Yann LeCun, one of the founders of the current artificialintelligence revolution and a recipient of the Turing Award, the equivalent of a Nobel Prize in computer science, in 2018. He is vice president and chief AI scientist at Facebook.
Methods that do not rely on such precise human-provided supervision, while much less explored, have been eclipsed by the success of supervised learning and its many practical applications — from self-driving cars to language translation. But supervised learning still cannot do many things that are simple even for toddlers. “It’s not going to be enough for human-level AI,” said Yoshua Bengio, who founded Mila, the Quebec AI Institute, and shared the Turing Award with Dr. LeCun and Geoffrey Hinton. “Humans don’t need that much supervision.” Now, scientists at the forefront of artificial intelligence research have turned their attention back to less-supervised methods. “There’s self-supervised and other related ideas, like reconstructing the input after forcing the model to a compact representation, predicting the future of a video or masking part of the input and trying to reconstruct it,” said Samy Bengio, Yoshua’s brother and a research scientist at Google.
There is also reinforcement learning, with very limited supervision that does not rely on training data.
Reinforcement learning in computer science, pioneered by Richard Sutton, now at the University of Alberta in Canada, is modeled after reward-driven learning in the brain: Think of a rat learning to push a lever to receive a pellet of food. The strategy has been developed to teach computer systems to take actions.
Craig S Smith hosts the podcast Eye on AI and is a former correspondent for NYT© 2020
The New York Times
Visit news.dtnext.in to explore our interactive epaper!
Download the DT Next app for more exciting features!
Click here for iOS
Click here for Android